Unlocking the Enormous Potential of TinyML for Modern Tech
Written on
Introduction to TinyML
TinyML is rapidly emerging as a transformative technology, harnessing the power of low-energy microcontrollers for machine learning applications. This innovation opens up a plethora of opportunities that were previously unimaginable.
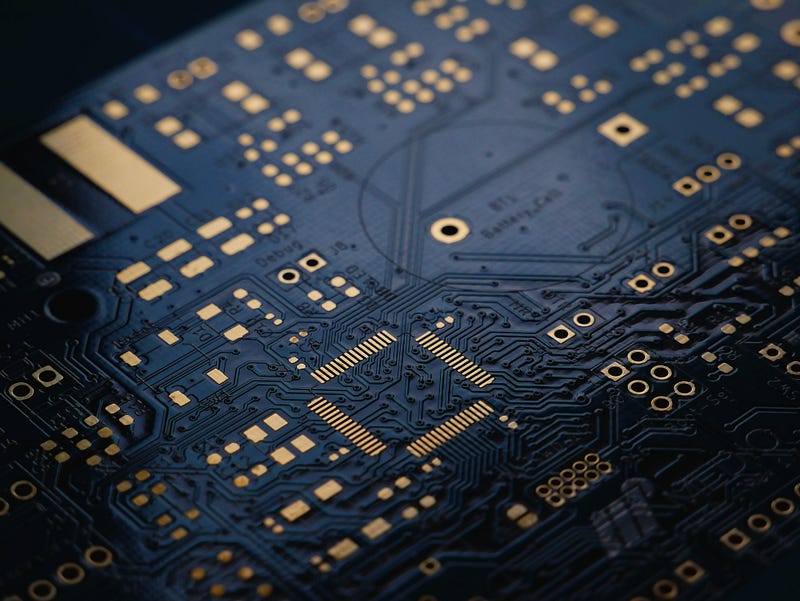
Photo by Vishnu Mohanan on Unsplash
Machine learning serves as a pivotal force driving many of the 21st century's groundbreaking advancements. Each day, we engage with machine learning models that simplify our lives, from personalized social media feeds to accurate weather forecasts and navigation assistance. The ongoing evolution towards the Internet of Things (IoT) necessitates increasingly advanced ML models for seamless technological integration.
However, a significant hurdle in machine learning remains its high computational cost. Currently, only large data centers equipped with powerful computing resources can effectively develop and train these sophisticated models. This scenario raises challenges, particularly regarding latency, bandwidth, and the centralization of power among major tech corporations.
Yet, within this landscape lies a tremendous, often overlooked opportunity: microcontrollers capable of executing deep learning algorithms. Though microcontrollers, such as those found in remote control systems, have existed for some time, advancements now allow them to perform intricate machine learning tasks.
With enhanced hardware and improved development practices, programmers can create applications for microcontrollers. For instance, voice-activated services like “Okay Google” and “Hey Siri” utilize modified deep learning models that operate on these devices. This evolution has given birth to the field of Tiny Machine Learning, or TinyML.
What is TinyML?
TinyML represents a form of machine learning that condenses deep learning networks to fit within compact hardware. It occupies a unique space at the crossroads of embedded systems and machine learning, enabling complex ML models to run on ultra-low power microcontrollers. This capability allows deep learning algorithms to train directly on devices, eliminating the need to transfer data to cloud services, a process known as edge computing.
Edge computing is invaluable. While IoT has transformed the utilization of embedded devices, transmitting sensor data to the cloud for processing is not always practical due to constant power and connectivity demands. Edge devices are designed to execute specific tasks based on machine learning models independently of cloud services. TinyML leverages affordable 32-bit microcontrollers to implement ML models on these edge devices.
The outcome is a new breed of smart embedded systems that can be deployed anywhere without reliance on cloud servers, enabling us to utilize deep learning algorithms to enhance product quality and performance.
The Expansive Potential of TinyML
For years, leading tech companies like Apple and Google have kept the synergy between deep learning and microcontrollers largely to themselves. However, the TinyML movement is now gaining traction.
The benefits of TinyML systems over traditional IoT solutions are numerous:
- Low Latency: TinyML enables local processing of data without requiring server communication, significantly reducing output latency.
- Reduced Bandwidth: With less frequent data transmission to servers, the demand on internet bandwidth is minimized.
- Enhanced Data Security: Since the model operates locally, sensitive data is not stored on potentially vulnerable servers.
- Minimal Power Consumption: Microcontrollers consume very little power, allowing them to function for extended periods without recharging.
Applications of TinyML
TinyML's capability to analyze and summarize data on edge devices is revolutionizing various industries. As the global trend shifts towards seamless technology integration via IoT, TinyML may emerge as a key player due to its low bandwidth and latency requirements.
Healthcare
Digital health monitoring is one of the latest advancements in healthcare. By utilizing machine learning models on endpoint devices, healthcare professionals can track vital diagnostic patterns across various patient parameters, enhancing predictive analytics and improving personalized care.
Real-time Analytics
TinyML is crucial for audio analytics, pattern recognition, and voice human-machine interfaces. This data can be leveraged for diverse applications, including elder and child care, safety, and equipment monitoring.
Mobility and Traffic Management
TinyML can facilitate real-time traffic monitoring and control, improving city mobility and enhancing the efficiency of autonomous vehicle sensors. Could TinyML also play a role in addressing ethical dilemmas like the Trolley Problem in autonomous driving?
Manufacturing
In manufacturing, TinyML can help prevent equipment failures by alerting workers to perform maintenance based on real-time equipment conditions. This technology can accelerate the development of smart factories.
Agriculture
Farmers worldwide face considerable risks from pests and diseases. TinyML can enhance agricultural efficiency and health, with applications like livestock wearables that monitor vitals to predict illnesses.
Getting Started with TinyML
The Arduino Nano 33 BLE Sense serves as an excellent platform for running machine learning models on edge devices. This microcontroller possesses the necessary capabilities for TinyML applications. TensorFlow Lite is the leading ML framework, continuously evolving to enhance its features.
As a nascent field, TinyML currently lacks extensive learning resources, but beginners can explore materials such as Harvard University’s course on TinyML and Pete Warden’s book, TinyML: Machine Learning With TensorFlow Lite on Arduino and Ultra-Low Power.
The Future of TinyML
The future of TinyML is incredibly promising, with expectations for significant industry growth in the coming years. The surge in microcontroller sales can be attributed to the IoT trend, with over 250 billion active embedded devices projected to grow by 20% annually, according to McKinsey & Company.
The COVID-19 pandemic has further accelerated the development of IoT systems, many of which incorporate TinyML for remote data analytics. This momentum is expected to continue amidst fierce industry competition.
Ultimately, TinyML has the potential to democratize access to vital machine learning tools, reducing the costs associated with running algorithms. This shift will enable the transformation of wasted data into actionable insights, fostering the growth of various industries.
While we are still in the early stages of TinyML adoption, further advancements are necessary for mainstream integration. Increased investments in open-source projects supporting TinyML, such as Google’s enhancements to TensorFlow, are crucial. Collaboration from chipset manufacturers, cloud providers, and dataset managers will also be vital for accelerating TinyML's growth.
Innovation drives change in every industry, and embracing new paradigms is essential. The future of machine learning is undoubtedly becoming smaller, and TinyML stands at the forefront of this evolution.
TinyML Talks: Introducing Wake Vision – A New Era in High-Quality Datasets for Tiny Machine Learning
This video discusses how Wake Vision is setting a new standard in creating high-quality datasets specifically designed for TinyML applications, enabling more effective machine learning on low-power devices.
TinyML TALKS: Is it time to take control of your Edge AI Project?
In this video, experts discuss the importance of managing Edge AI projects effectively, highlighting the potential benefits of TinyML in various applications.